From Stochasticity to Functionality
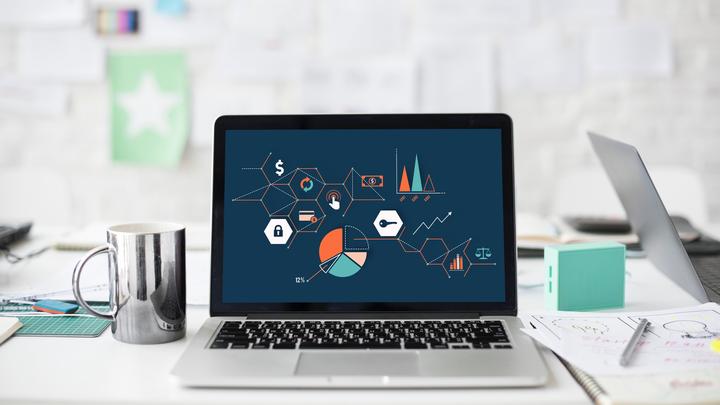
Machine learning, or the ability of computers to intelligently analyse complex data sets, may be the defining technology of our age. Society produces huge amounts of data in areas as diverse as economics, weather, medicine, science and social media, analysis of which can greatly enhance our decision making. However, current computers are poorly suited to analysing large and complex datasets, particularly when compared to animal and human brains.
For example, while a typical contemporary computer can perform simple numerical calculations much more quickly than the brain, its efficiency is almost one million times lower when performing more complex data analysis such as recognising human faces. This inefficiency results from attempts to emulate “neuromorphic”, or brain-like, computational processes by brute force on hardware which is ill-suited to the task. For example, in a conventional computer memory and processing are inherently separated, whereas they share the same medium in the brain. To overcome these limitations, bespoke computers that are specifically designed for neuromorphic computation are required.
Away from pure computational power, the “big data” revolution has been driven by our ability to store information. This is the result of nano-scale magnetic technology in the form of hard-disk drives. However, nanomagnetic devices suffer from an encroaching limitation, in that their behaviour becomes increasingly unreliable, or stochastic, as they are further miniaturised to increase performance. While this is devastating for conventional digital computers, there is strong evidence that stochastic behaviour can be tolerated in, or even enhance, the performance of neuromorphic technologies. This raises the tantalising prospect of nanomagnetic technology being ideally suited for developing new computer forms of computer hardware for data analysis.
In this project, a collaboration between experts in nanomagnetic technology and computer science at the University of Sheffield, we will perform a pilot study to investigate whether stochastic behaviour in “magnetic domain wall devices” a promising form of magnetic nanotechnology where magnetic information is flowed through nanowire conduits, can be used to realise new, neuromorphic computer architectures. Working with an advisory board of academic and industry experts in machine learning technology we will demonstrate new data analysis devices, before creating a roadmap to develop the devices into real technology. Eventual success in developing these technologies could result in powerful hardware platforms that can provide even the smallest device the ability to intelligently analyse its environment to make informed decisions.
Credit: Tom Hayward, Dan Allwood and Eleni Vasilaki